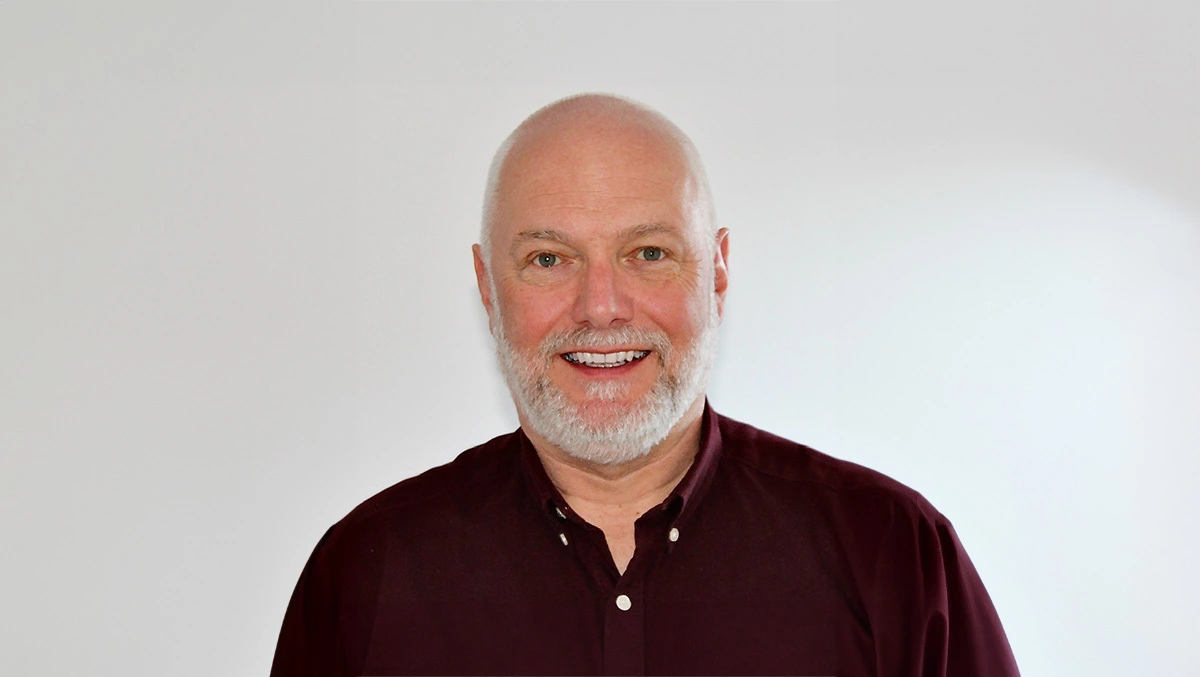
Why the answer to AI threats is not more AI
AI is not new – quite the opposite; in the tech world, we have heard of little else in recent years. When it comes to AI in cybersecurity, AI is casting doubt over existing security tools, and the current concern is that as it can be used to facilitate the evasion of these tools. AI-based attacks can easily find vulnerabilities and open and exploit ports to run malicious code or move to high-value assets.
A question many in the industry are asking themselves is, should we fight fire with fire? Recent research from KnowBe4 has shown that close to half of IT decision-makers in Singapore believe the use of AI to combat cyber threats is one of the most beneficial ways to protect their organisations. However, in this article I'll cover some of the latest emerging AI attack trends, and why the answer to AI threats is not more AI.
Emerging AI threats and tactics
One very effective tactic we've seen is 'phone home morphing.' Once malware has successfully penetrated the target network, this technique uses an API to call back to an AI tool to report its progress and receive updates to help it move forward. So ransomware blocked by an Endpoint Detection and Response (EDR) tool will 'phone home' to explain what stopped it and then receive an update on how to overcome the obstacle. This happens repeatedly until the malware succeeds.
Even more dangerous than this is the concept of a self-generating polymorphic code. Rather than calling back to base, this AI malware can learn from its environment independently and adapt its tactics to "live off the land" and progress its attack. This approach is currently too resource-intensive to be viable, but it's only a matter of time as computing power advances.
Alongside the threats from AI, there are also threats to AI, known as AI poisoning. This is where bad actors manipulate AI tools that learn from information to identify patterns and trends. By poisoning this data with false information, it's possible to trick AI into learning the wrong lessons. This could mean deceiving systems into thinking malicious activity sets are benign, enabling attackers to go unnoticed.
Fighting fire with fire is not the right approach
Combatting AI threats with AI has become one of the most common responses to emerging threats. What better way to counter an inhumanly fast threat than with an equally dynamic, defensive AI? But while AI undoubtedly has its place in the security tech stack, relying entirely on this approach to combat new threats is a mistake. The ability for adversaries to poison and subvert defensive tools means that there's always a risk that AI-powered security solutions will be tricked into overlooking malicious activity.
Wider deployment of AI threat detection means more opportunities for threat actors to understand how tools work and counteract them. As such, AI should be used judiciously, just as we use antibiotics with caution to bring about the greatest effect when fighting infection. The best strategy is to limit the impact of AI-powered attacks by tightly controlling the environment they can access.
Reduce AI's ability to learn
Reducing the attack surface is already a mainstay security strategy for keeping attackers out. Now we also need to think in terms of limiting the "learning surface" available to offensive AI tools already within the network. Blocking invasive malware from accessing resources means the AI behind it will have less opportunity to learn, adapt, and progress the attack.
One proven strategy for doing so is breach containment. This focuses on limiting and containing how malicious actors can spread through the network using Zero Trust Segmentation, also known as micro segmentation. Rather than trying to outpace and catch an intruder, the threat is halted in its tracks until it can be eliminated. This has a knock-on effect of improving incident recovery as the impact radius of an attack is far more limited.
The problem is traditional network segmentation approaches do not provide the control and agility needed to fight AI-powered threats. They offer no ability to change security rules per asset, based on status and context, which makes it increasingly difficult to keep up.
In order to come up against the burgeoning AI threat, we need a step change in security – one that moves away from the static, network-based cybersecurity approaches of the past to a more dynamic approach that applies security controls on a much granular level based on risks identified. We must restrict the ability of an AI attack to learn about the defences and systems, thus reducing the effectiveness of any attack. Using a more dynamic approach, organisations can respond and recover more quickly in the event of any AI-powered breach without having their systems shut down in the interim.