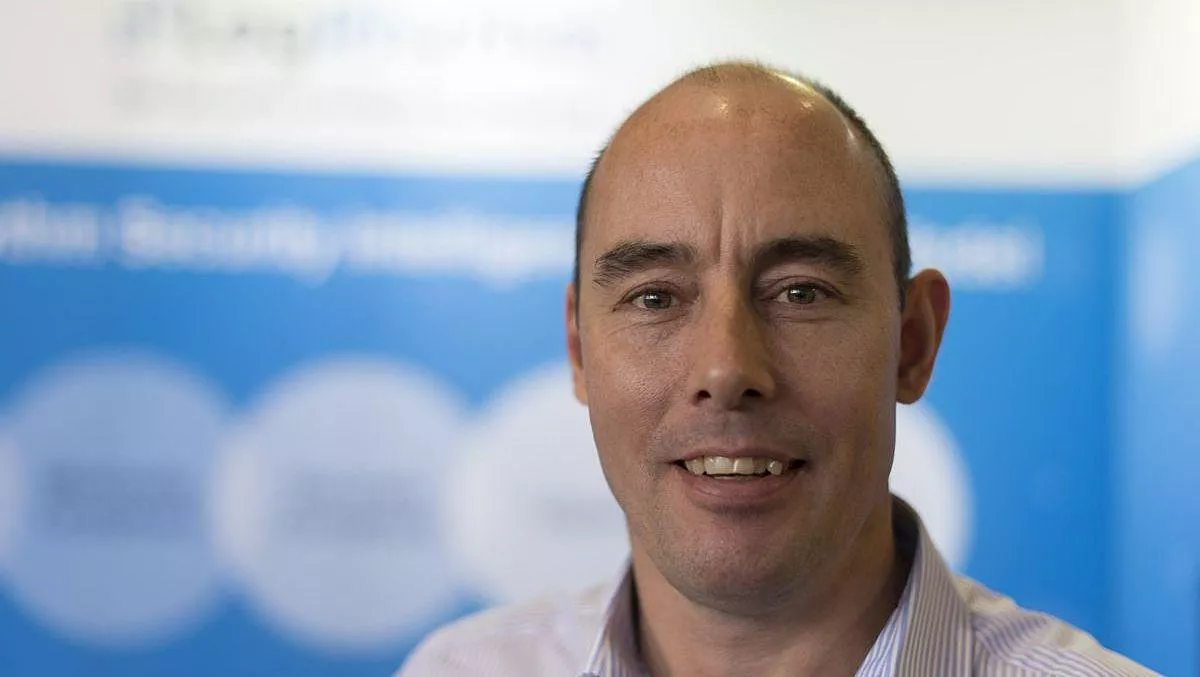
Why AI and ML are increasingly important for effective IT security
With the evolving capabilities of artificial intelligence (AI) and machine learning (ML) attracting increasing interest, attention is being directed at how they can benefit IT security. Both vendors and customers are examining ways in which the technologies can strengthen defences and ward off attacks.
From a security professional's perspective, the need for AI and ML is strong. They're looking for ways to automate the task of detecting threats and flagging malicious behaviour. Moving away from manual methods will free up time and resources to focus on other tasks.
The challenge is exacerbated by the huge numbers of false positive reports generated by many current security monitoring tools. Teams struggle to keep up with the activity to be analysed, or find they simply can't identify emerging threats amid the noise.
The power of AI and ML
This is where AI and ML can deliver real value. ML offers much better capabilities than humans can deliver when it comes to recognising and predicting certain types of patterns. These new tools can also move beyond rule-based approaches that require knowledge of known patterns. Instead, they can learn typical patterns of activity within an IT infrastructure and spot unusual deviations that could mark an attack.
However, while modern tools such as AI and ML can support a CISO's arsenal of cyber support infrastructure, organisations still require some human involvement to respond and recover from incidents. For example, in areas such as deciding if an issue is a false positive, communicating with the affected team, and coordinating actions with other organisations.
Indeed, today's security products cannot fully automate the Security Operations Centre (SOC) and completely eliminate the need for security analysts, incident responders, and other SOC staff, but technology can streamline and automate some process to reduce the need for human responders.
ML itself offers a number of ways to improve an organisations infrastructure security. These include:
- Threat prediction and detection, where anomalous activity is assessed in order to recognise emerging threats
- Risk management, involving the monitoring and analysing of user activity, asset contents and configurations, network connections, and other asset attributes
- Vulnerability information prioritisation, by using learned information about an organisation's assets and where weaknesses might exist
- Threat intelligence curation through which information within threat intelligence feeds is reviewed to improve quality
- Event and incident investigation and response, which involves reviewing and analysing information on events and incidents in order to identify next steps and organise the most appropriate response
AI and UEBA
Another area in which these emerging technologies can assist security teams is in user and entity behaviour analytics (UEBA). User and entity-based threats are a growing concern and new approaches are needed.
According to a recent Verizon Data Breach Incident Report, 63% of confirmed data breaches involve attackers posing as legitimate users by using stolen access credentials, or legitimate users maliciously exploiting their access.
However, to detect insider threats, security tools must first be able to understand and baseline user behaviour, and this is where ML can provide real value. By establishing baseline behaviours and patterns, then detecting anomalies by combining statistical models, ML algorithms, and rules, a UEBA solution can compare incoming transactions with the existing baseline profile. Potential threats can be flagged for further examination and action.
Specific areas in which AI can assist with UEBA include:
- Account compromise: The AI-powered tools can detect whether a hacker has accessed a network user's credentials, regardless of the attack vector or malware used
- Insider threats: By establishing baseline user behaviour, the tools will be able to detect and flag unusual, high-risk activity that falls out of that baseline
- Privileged account abuse: An AI-assisted UEBA solution will identify specific attacks on privileged users who have access to sensitive information by detecting compromised credentials and lateral movement to the systems that contain this privileged data
Ongoing improvements to IT security
Together, AI and ML technologies have a lot to offer security teams looking for better ways to protect against and respond to cybersecurity threats.
However, to achieve all that the technology has to offer, security teams will need to be mindful of some key steps that have to be taken. These include:
- Providing ML-powered tools with real-time access to large sets of high-quality, rich structured data that shows all security-related events throughout the organisation
- Feeding the tools with the contextual information necessary to understand the meaning and importance of each observed activity and detected anomaly
- Performing supervised learning with extensive sets of high-quality training data to educate the tools on which activities are good and which are bad.
Deployed and managed well, AI and ML-powered tools will offer significant support and assistance improvements for security teams. They will detect hidden threats and minimise false positives, accelerate incident response and streamline the running of the Security Operations Centre (SOC), thereby reducing costs and improving efficiently.
The evolution of AI and ML has only just begun and its capabilities will continue to accelerate in coming years. It's worth taking the time know to understand the technology's capabilities and exactly how it can add value to your organisation.