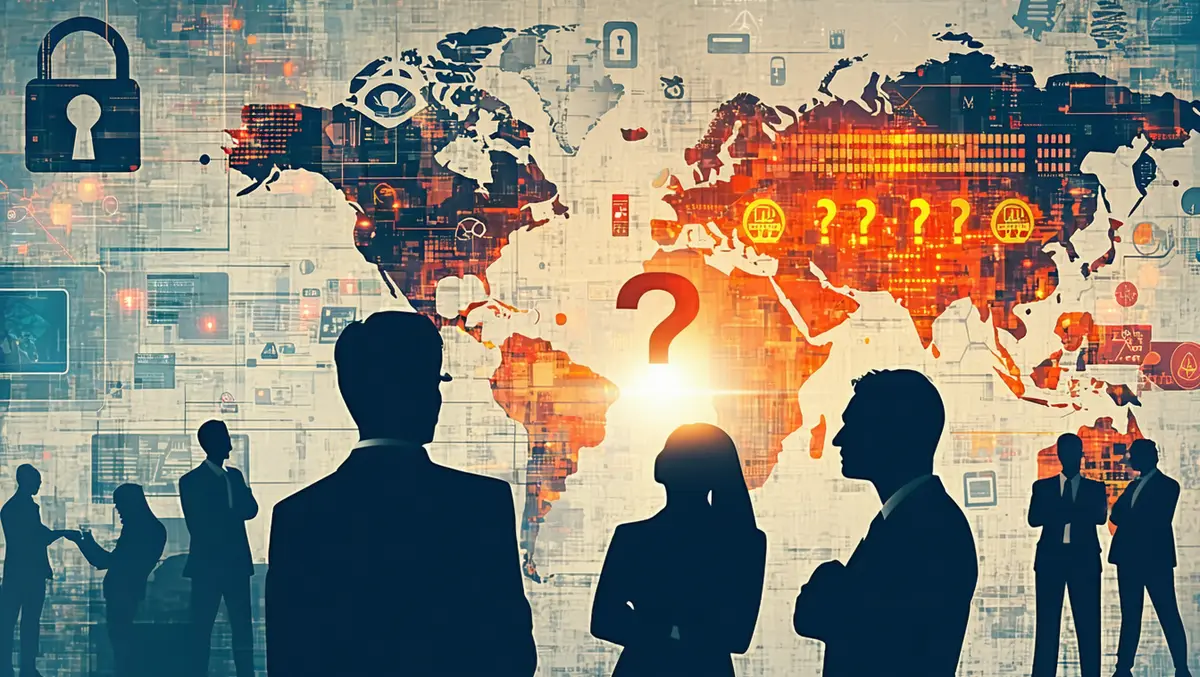
DeepSeek models spark global regulatory & privacy debates
The large language model (LLM) known as DeepSeek has rapidly gained attention following the release of its newest models, DeepSeek V3 and DeepSeek R1, prompting discussions around security, privacy, and potential regulatory issues.
Tenable's Security Response Team (SRT) has published a detailed Frequently Asked Questions (FAQ) document aiming to address prevalent questions about DeepSeek, shedding light on its operational framework and examining the implications for enterprises considering its adoption.
The document provides background on DeepSeek, which was founded in 2023 by Liang Wenfeng. The FAQ describes LLMs as machine-learning models pre-trained on extensive data corpora, allowing for natural, human-like responses.
Interest in DeepSeek has surged due to the models being open-source, allowing individuals to run them locally, and their reportedly less demanding hardware requirements. This development signifies a lower-cost approach to model training, which has been noted as significant within the industry.
DeepSeek V3 utilises a mixture-of-experts (MoE) technique, functioning with reduced computational power by loading only necessary "experts" for responding to prompts. It also incorporates a method called multi-head latent attention (MLA), which minimises memory usage and enhances performance during training and inference processes.
Additionally, DeepSeek R1 introduces a multitoken prediction (MTP) architecture, enabling the model to predict the next two tokens simultaneously. This model also features a reasoning approach including chain-of-thought (CoT), showcasing the reasoning process for user interactions.
The FAQ outlines the system requirements for running DeepSeek models locally, indicating that models with 1.5 to 70 billion parameters can be operated on consumer-grade hardware. The model requirements scale with parameter size, with larger models necessitating more advanced hardware.
Benchmark comparisons show that DeepSeek R1 competes with established models from OpenAI, Claude, and Meta. Notably, unlike other industry models, many of which remain proprietary, DeepSeek is open-source, providing accessibility advantages.
The document also addresses safety concerns, noting that the open-source version of DeepSeek potentially mitigates risks better than its website or mobile applications, given the absence of internet connectivity requirements. Privacy issues arise from data collection practices detailed in DeepSeek's privacy policy, which states that user data is retained on servers located in China. The lack of alignment with international privacy standards, such as the EU's GDPR, has raised concerns.
Further regulatory scrutiny is noted as several countries and U.S. states have implemented or are considering bans on DeepSeek due to privacy and security concerns. These include Italy, Taiwan, South Korea, Australia, as well as U.S. states such as Texas and New York and federal departments such as the Department of Defense and the U.S. Navy.
The FAQ concludes with Tenable's ongoing investigations into LLM safety and security issues, with commitments for future updates to be shared through its publication platforms. Satnam Narang and Nick Miles authored the FAQ, bringing extensive expertise in cybersecurity and machine learning to the forefront of the discourse on DeepSeek.