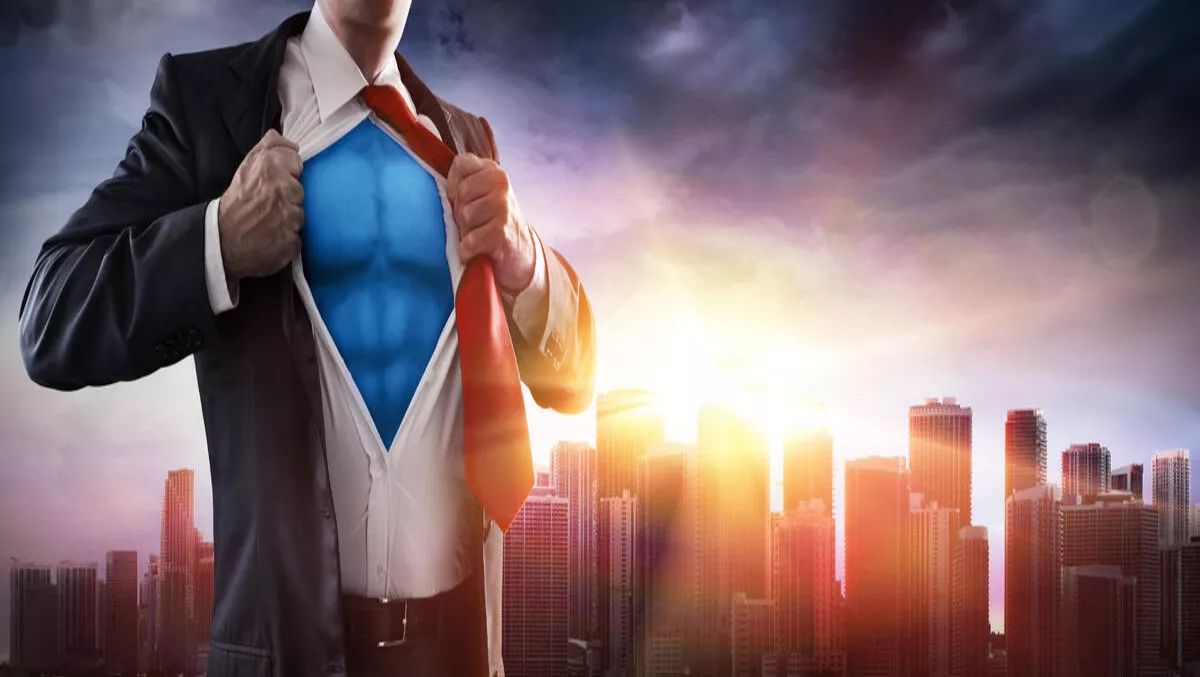
Artificial Intelligence: Out of the futurists’ lab, into the real world of networks and cybersecurity
Artificial Intelligence to the rescue! AI is widely seen by scientists, vendors and even enterprise IT professionals as the next step in cyber defense. It's a perfect match. Cyberattacks are coming faster than humans can respond – and are morphing into new dangers that traditional anti-malware software can't always detect. That's where AI's self-learning capabilities excel, and are able to respond at the speed of light (or at least, at the rate of high-speed networks and modern microprocessors) in an adaptive, effective fashion.
We recently spoke to three cybersecurity experts to get their take on the state of AI for next-generation network defense – and their views about the future: Kathryn Hume, President, Fast Forward Labs; Stuart McClure, Author, Inventor, and CEO, Cylance; and Paul Jackson, Principal Analyst, Digital Media, Ovum.
Kathryn Hume explained that artificial intelligence algorithms always start with particular use cases and particular data sets "from which we elicit general algorithms that then may or may not be able to be applied to different use cases but both the opportunity and the complexity of this space lies within that transition from particular to general."
For example, she cites the well-known IBM Watson computer that won on the game show Jeopardy by focusing on a specific data set; "we've seen Google DeepMind build AlphaGo which is a tool using a technique called reinforcement learning, a set of artificial intelligence algorithms that put in position a system of rewards to train systems to excel at a particular task." In that case, AlphaGo developed and used a set of algorithms to beat Lee Sedol, the leading Go champion, in March 2016.
From Fun and Games to Data and Networks
Beating humans at trivia and at board games is one thing; it's great for building awareness of AI and of exciting the popular press, but it doesn't apply directly to enterprise computing. Neither do other applications of AI that we encounter as consumers, such as Facebook image recognition or textual analysis of Twitter posts to learn about users' political preferences. How about protecting computer networks from attackers and malware? It's all the same problem, said Ms. Hume: Studying huge amounts of training data to learn patterns – and then rapidly searching for those same patterns in real-world situations.
Cylance's Stuart McClure picked up the narrative, explaining that for software that monitors the data stream – such as network traffic or email attachments – the goal is to quickly determine if the traffic or file is safe or malicious. That requires a lot of deep learning to see patterns – and the ability to evaluate new data quickly, to see if it meets the patterns of safe or malicious.
Mr. McClure used the analogy of watching a house to determine if a person walking nearby is a burglar. "Some cybersecurity platforms cannot determine if something is bad unless they've seen it before and know what it will do. It's sort of saying, well I can't tell if this person walking up to my house is going to burglarize it until they actually break in and steal something. Right? That's not very effective.
By contrast, there's a better, more effective way, said Mr. McClure, which is to learn patterns – and not trust anything suspicious. "If you were to watch the video cameras from every home around the world, for every burglar that ever walked up to a house and burglarized it you'd create patterns in your mind. You would create connections between how they were dressed, how they approached the house, how they interfaced with the locks. You would figure it out pretty quickly if they were good or bad. So when a new person came up to your house you'd think, uh this person matches the pattern for a burglar. I'm not going to trust them. I'm going to watch them the whole time.
The Cybersecurity AI Breakthrough
Mr. McClure applied that reasoning to cybersecurity where, in the old, pre-AI model, an anti-malware company needed thousands of analysts to write rules and virus signatures, by studying malware that evaded previous rules and signatures. "That's not scalable," he said, and can't reach the 99% success threshold needed for effective protection. "We can't possibly scale like that with thousands of analysts writing rules and signatures. The threats come out way too fast.
That's when Mr. McClure, through Cylance, had the breakthrough: Instead of studying the latest malware to write new rules and signatures – and therefore, detect it only after it successfully attacked someone – why not use artificial intelligence?
"That's what we've been able to do," said Mr. McClure. "We talk about two parts of AI quite a bit - supervised and unsupervised learning. There are two parts to what we do. The first part is we automatically look for features that are going to be potentially indicative of good or bad." That's not just a few features, by the way - not even just a hundred features. "Now if I told you we have over five million features that are indicatively defined as malicious or safe you probably wouldn't believe me. Right? Five million? That's insane.
The first part is to use software to look for features that might indicate malicious intent in a file. The second part? A supervised human judgment of whether sample files are actually malicious or not. "We collect as many files as humanly possible. Then we extract as many features as we possibly can that we've already mapped or learned are potentially useful. Then we transform those. We then train the AI using neural networks about what is going to cluster to good and what is going to cluster to bad. Then we classify it. If it's bad we block it. If it's good we allow it. It's that simple.
Ovum's Paul Jackson observed that while AI has been around for decades, both in the lab and in commercial products, there have been many rapid advancements recently. "To a lot of us, practical AI seems to have really come to the forefront over the last 12 or 15 months, butwhy now?
Fast Forward's Ms. Hume agreed with that point: many techniques such as neural networks and deep learning have been around since the 1990s, and in some cases AI goes back to the 1940s. But there were some problems, she said, and some tools that didn't yet exist. "There wasn't a lot of data to work with. We didn't have the big data area - I use the term big data to refer to storing and processing data, not doing stuff with it. So 10 years ago it became really cheap to store a lot of data, keep it up in the cloud and then do stuff with it.
Indeed, when it came to practical pattern recognition, she continued, "Around 2011 was when Google had a first coup using artificial neural networks to automatically identify cats in videos across the Internet. Computers needed to figure out that there was something about cats that made them similar, and could cluster together all these patterns. Then the supervised part was humans coming in and saying, oh yeah that thing you see that looks kind of like a blob of something, this amoeba thing, that's called a cat. And that one isn't a cat.
The Rise of the GPU and Big Data
Another factor, Ms. Hume said: the rise of graphical processing unit (GPU) chips that excelled at pattern recognition processing. "Some kid playing video games realized that the structure of GPUs to process images were pretty good at matrix multiplication, which just so happens to be the type of math that's powering these deep learning algorithms. So they said, the gaming industry is huge but gosh this other thing might be a lot bigger if we can actually apply these things to enterprise artificial intelligence needs, and this lets us train those neural networks faster.
"Another factor in AI's rapid rise is the data," added Ms. Hume. "It takes a neural network probably 50,000 examples in order to gain that ability to recognize things. So you can imagine if we're going to go through all of the types of objects we might want to identify to build a recognition system we need a lot of training examples. So that data has also propelled the transition.
Cylance's Mr. McClure cited a fourth breakthrough technology: Cloud computing. "We never could have started this company and done what we've done without the cloud, without Amazon Web Services in particular. Two or three years ago, it would literally take about six months to build a malware detection model. Today our models take about a day and a half to build. But we have to spin up over 10,000 CPUs to do that in a day and a half. Without that flexible compute fabric there's no way we could be doing what we're doing. It's just that simple.
The Perfect Place to Apply Artificial Intelligence
Ovum's Mr. Jackson observed that, "We are increasingly facing many more sophisticated types of attack, and that end point protection is a key goal of cybersecurity systems. This type of security seems to be one of those areas where AI is particularly well suited, because trained tools can perform far better than people.
Cylance's Mr. McClure agreed that cybersecurity is the perfect place to apply AI and machine learning. "Quite honestly I don't know why it hasn't been done before! That seems pretty easy, straightforward. That would be a natural assumption to apply.
He continued by citing three core ways that attackers manage to penetrate systems, all of which can be blocked or mitigated through the use of AI:
"First: Denial of Service, which starves the resources of the target. So you starve memory,you starve network bandwidth, you starve a CPU or a disc or something and the system falls down. It breaks.
"Second: Execution based attacks, which is what Cylance protects against. An attacker gets something sent to you or gets you to click on something that executes something in memory to do malicious things on your computer.
"Third: Authentication based attacks. Being able to steal your password and pretend to be you on your computer when you're not there, or bypassing authentication or brute forcing your password or any of those things.
"AI can be applied to all three of those areas in a very meaningful way, you just need the data.
How about the Rise of the Machines?
Mr. Jackson looked into the future, and was playfully concerned about what he might see. "We have talked about unsupervised and supervised learning. There is a whole realm of fear around wholly unsupervised AI, a sort of ghost in the machine, like the Terminator's Skynet. The growth of AI is discussed a lot in the press - are those worries unfounded? Realistic? Is dangerous AI something we have to keep an eye on?
Fast Forward's Ms. Hume was not completely reassuring. "The thing to be concerned about in the near term is supervised learning, not unsupervised learning. That's not because computers are dangerous but because people are dangerous. Why? There are all sorts of things that we do as people in society. We leave traces of that in our data.
And, she continued, supervised learning requires human input and that input may not always be benign, or particularly thoughtful "We train systems based upon the decisions that humans have made in the past. So let's take an example of using algorithms to try to automatically hire somebody into your company or recruit students to your school or even give a loan for a credit application. If we try to automate that, the systems aren't that smart. They go out and they look in data sets. If in the past a specific university tended to recruit a certain type of candidate, the system will make future decisions based on that data. If the university tended to recruit relatively wealthy white males, the AI will build a model based on those past decisions.
That can lead to perpetuating those decisions – without any specific intent to do so, Ms. Hume continued. "We go into the system and we say here is a model for the type of candidate we're looking for. These are the decisions that humans have made in the past. The algorithm will then learn to find candidates that look like those, basing its decisions upon what the humans did. The result? The AI algorithm comes back and says, 'here is a pool of 95 per cent rich white males that we suggest you recruit to your school, precisely because if we think about a normal distribution this is where the bulk of the features tend to lie.' "
Ms. Hume concluded, "If we relegate our decisions to the algorithms they tend to propagate and amplify the stupid decisions we as humans have made. It's not about systems being stupid or intelligent, it's about our mixing together the corporate values with social values. We as data scientists may take an ethical position with regards to potentially having to hack the AI-learned algorithm so that we can create the future that we want, instead of one that perpetuates our biases from the past.
Look Out, Ransomware, Here Comes AI
Cylance's Mr. McClure closed the conversation with an example of using AI algorithms to classify and defend against one of this year's biggest challenges: Ransomware. It's a numbers game, he said – the more effective AI is in blocking ransomware, the less attractive sorts of attacks will be.
"We are seeing effective defenses against ransomware today," he said. "With the AI technology that we have installed on over three million end points, we already have the ability to have all of that technology truly detect malware and get to the ninety-ninth percentile of protection, and that includes about 350,000 to 400,000 new attack variants that come out every day.
As advanced AI-based malware detection tools deepen their market penetration, Mr. McClure added, cybercriminals will see that "all their new fancy attacks are no longer bypassing the security systems they are targeting. They are now getting caught. They're getting prevented. So there will be a natural desperation motivating the attacker to proliferate even more attacks.
Unfortunately for the attacker, that won't work, said Mr. McClure. "When attackers realize that doesn't work, they will get more sophisticated and spend a lot of money on trying to bypass the AI. I don't mind them bypassing us - I would actually love it because every single attempt to bypass helps us to make the AI model smarter.