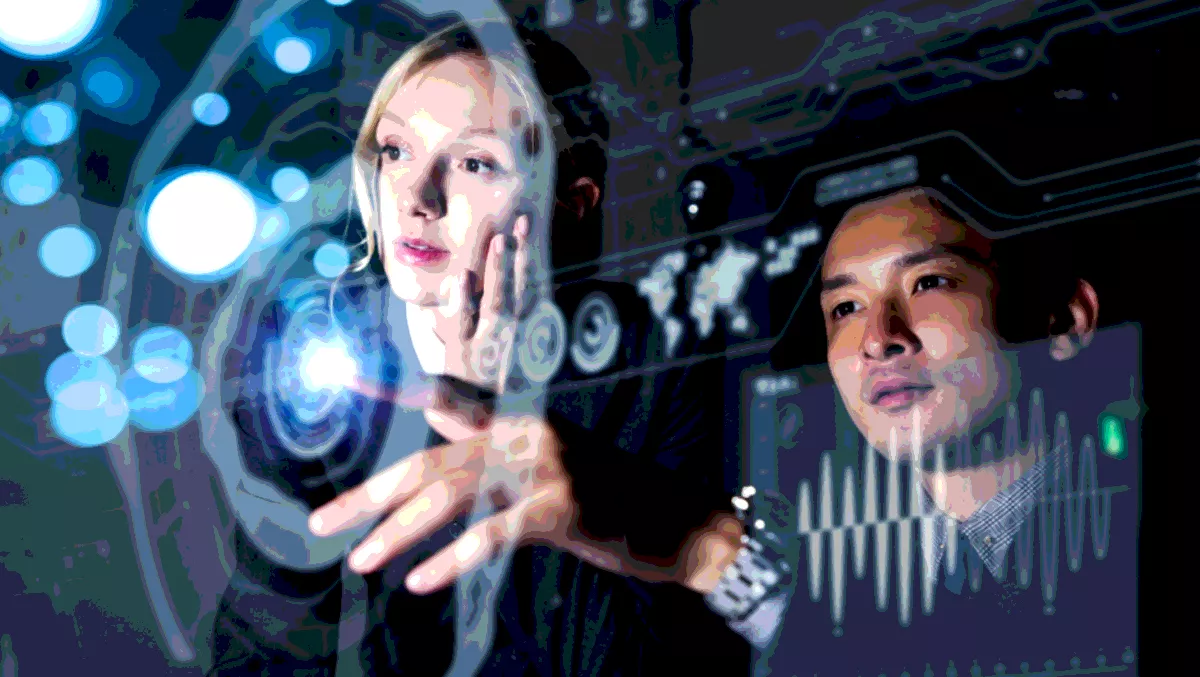
AI in cybersecurity stamping out menacing fileless attacks
With the storm of recent information and commentary regarding artificial intelligence (AI), specifically as it applies to cybersecurity, it can be difficult to understand how AI – or more specifically, machine learning (ML) and algorithms – are actually augmenting endpoint protection (devices ranging from servers to phones and IoT devices) for individuals and organisations.
Fundamentally, the difference between traditional and AI-based cybersecurity systems is a matter of "teaching" versus "learning".
Older antivirus software has been programmed to recognise specific security threats – phishing emails or ransomware, for instance – based on heuristics and specific digital signatures.
Software has been instructed on exactly what to look for as it scans through files. Of course, this means it's powerless against new threats that it doesn't recognise, which is why the system's maintainers are constantly adding new instructions to reflect the latest cyber threats.
You know those notifications you constantly get reminding you to update your antivirus software? That means programmers have updated the system to protect a newly discovered vulnerability (which is why it's important to keep your antivirus up to date).
Simple enough. At least it was for most of the internet's history. The problem with the traditional approach is that in recent years cyber threats have become too numerous and too sophisticated for legacy antivirus to keep up.
For example, in 2017 experts discovered over 7.4 million new malware specimens, leading some to call it the "Nightmare Year" for cybersecurity. To put that figure in perspective, it represents a 5,600 percent increase over the past decade.
Thankfully, recent advancements in machine learning – the building of algorithms that use statistical techniques to improve their own code – are enabling a more effective approach for endpoint security.
Rather than trying to "teach" software all of the individual threats to look for, engineers can instead feed machine learning algorithms millions and millions of examples of cyber threats, allowing the systems to "learn" for themselves how to distinguish friend from foe.
Thus, when hackers produce new variants of viruses and malware to avoid the signature-detection of traditional antivirus, machine-learning based endpoint security, understanding the patterns and anomalies that characterise malware, is rarely fooled.
One recent study found that an AI-powered system identified zero-day threats correctly 98.88 percent of the time, compared to the 71.16 percent success rate of traditional AVs.
So, does this mean machine learning is the silver bullet of endpoint protection? Not quite. The technology has limitations, most notably that it takes enormous amounts of data and computing power to make it work properly.
But machine learning is so effective that even hackers are using the technology, training their programs to evade even the most sophisticated defenses. Increasingly, cybersecurity is becoming a matter of AI versus AI.
As HBR recently put it, "AI is the future of cybersecurity, for better or for worse.
One area in which AI security models are proving to be particularly effective is in defending endpoints against so-called "fileless" attacks.
These attacks were first identified as early as 2001, but they've only come to the forefront of cybersecurity recently. In fact, last year according to "The 2017 State of Endpoint Security Risk" report by the Ponemon Institute, fileless attacks where included in an estimated 77 percent of successful data breaches worldwide. In that same report, Ponemon reports that 35 percent of all cyber-attacks are fileless exploits.
So, what are fileless attacks and what makes them so successful at infiltrating endpoints? Most often, traditional malware infiltrates digital endpoints by stowing away in long term storage, trying to trick users into executing malicious files so that it can take control.
Fileless malware, on the other hand, circumvents the stowaway step by infiltrating an endpoint's memory directly. It does this by manipulating a system's registry – the database where the system's low-level settings are kept – acting as a kind of deviant administrator to steal information or disable key features.
Often, this means that no action is required by the user for the "non-malware" to take hold. Even more important, this property makes it extremely difficult for traditional antivirus software to detect.
Signature-based scans typically don't interact with an endpoint's registry at all. And even if the AV does come into contact with the malicious code, its built-in heuristics will only rarely identify the fileless threat.
The beauty of machine learning-powered systems is that they don't rely on signatures, heuristics, or traditional scans at all.
Instead, they apply sophisticated algorithms to vast amounts of information, continuously updating themselves to recognize threats, new or old, based on patterns in the data.
This makes them extremely effective at identifying and protecting against fileless attacks. In the era of fileless attacks, AI is by far the best approach for cybersecurity.